Overview
Visually Interactive Neural Probabilistic Models of Language
Hanspeter Pfister, Harvard University (PI) and Alexander Rush, Cornell University
Project Summary
Deep learning methods have been a tremendously effective approach to predictive problems innatural language processing such as text generation and summarization. However, these black-box modelscan be difficult to deploy in practice as they are known to make unpredictable mistakes that can be hard to analyze and correct. The proposed research will target visually interactive interfaces for probabilistic deep learning models in natural language processing, with the goal of allowing users to examine and correct black-box models through interactive inputs. Through co-design of models and visual interfaces we will takethe necessary next steps for model interpretability. We propose to develop new probabilistic models withuser "hooks" in the form of latent variables. These hooks will help further model examination and correction through visual interfaces. Achieving this aim requires active investigation into developing new deep learning models, new analysis techniques, scaling our proposed methods, and integrating them within a commonvisualization framework.
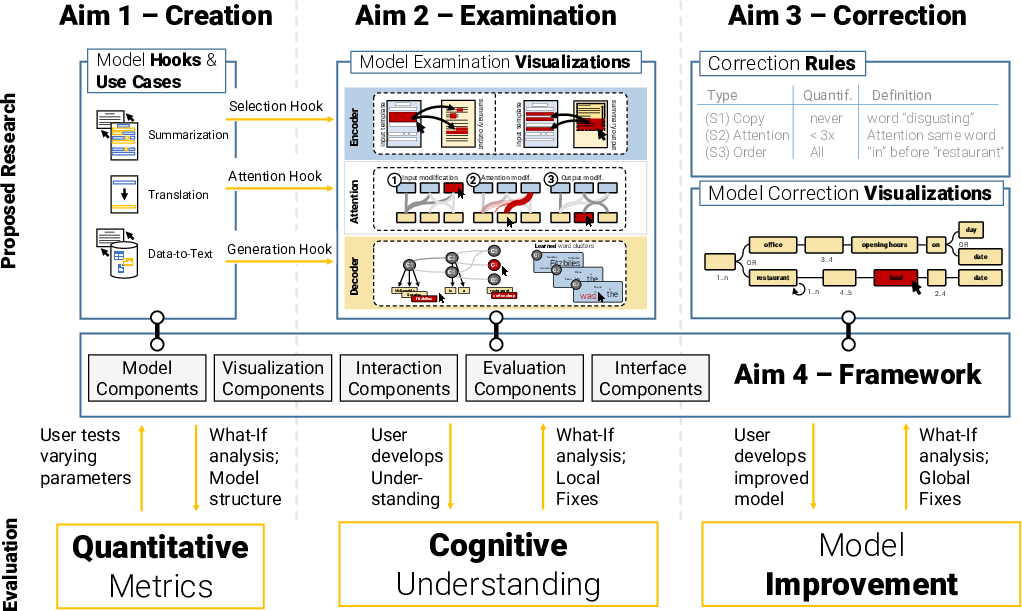